Deep learning virtual colorful lens-free on-chip microscopy
Download: 795次
Compared to the conventional microscope, lens-free on-chip microscopy whose principle is based on digital in-line holography has advantages of a further larger field-of-view (FOV), lower cost, and more compactness[1–
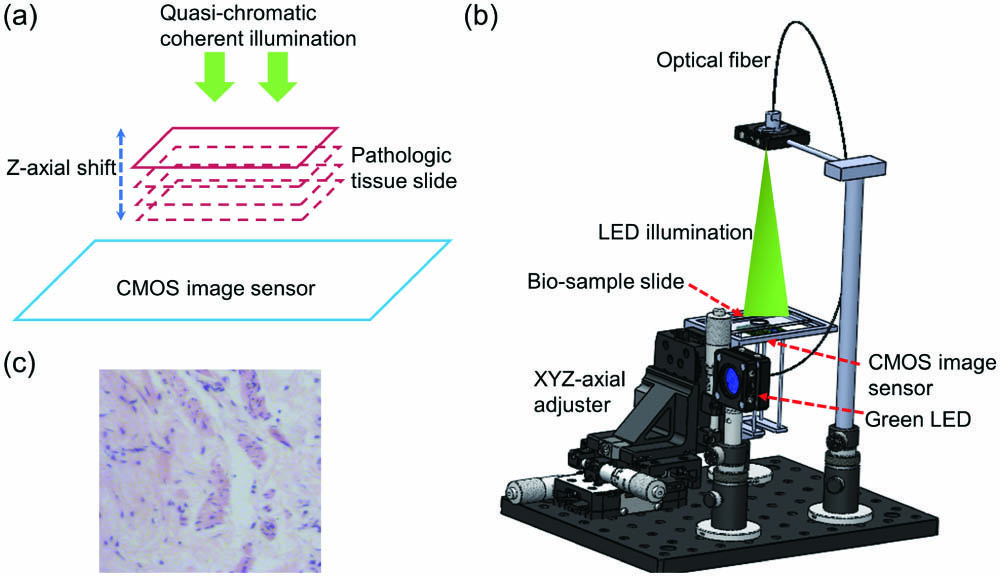
Fig. 1. Lens-free on-chip microscope setup. (a) Schematic of lens-free on-chip microscopy. (b) Experimental lens-free on-chip microscope setup. (c) An example image of H&E stained pathological tumor tissue.
In medical microscopy applications, chemical dye stained pathological human tissues are widely used. As shown in Fig. 1(c), hematoxylin and eosin (H&E) stain is one of widely used tissue stains in histology and medical diagnosis, which is viewed as a gold standard. For example, in a biopsy of a suspected tumor, the tissue is sectioned into micrometer-level thin slides, which are stained by a combination of H&E. Normally, the acidic structures of tissues and cell structures (basophilic) could be stained purplish-blue by hematoxylin dyes, which are basic dyes and positively charged. Hematoxylin is usually conjugated with a mordant (aluminum salt), which also defines the color of the stain. In order to form tissue-mordant-hematoxylin complex link, which can stain the nuclei and chromatin bodies purple, the mordant will bind to the tissue firstly, and then the hematoxylin will bind to the mordant. Eosin dye is an acidic dye, which is with negative charge (eosinophilic). Eosin stains the cellular matrix and cytoplasm (acidophil), giving them a red or pink color. In short, cell nuclei will appear purplish-blue, while the cellular matrix and cytoplasm will appear pink by H&E stain. Based on the two main colors, all structures of the tissue take on different shades and hues, which results in the general distribution of cells in the tissue sample, and its detailed structure can be easily observed. Consequently, it is easy to distinguish between the nuclei, cytoplasm, and boundaries of a tissue/cell sample in medical diagnosis by H&E stain.
The digital colorization of grayscale images is a hot topic in machine learning[5
In this paper, we propose a deep learning style transfer method to realize a colorful lens-free on-chip microscopy, with only one wavelength illumination. In theory, the data of our method is only 1/3 of conventional lens-free on-chip microscopy with RGB illumination, which means our microscope has higher efficiency and lower cost.
Lens-free on-chip microscope setup. Figure 1 presents our lens-free on-chip microscope setup. It mainly includes: 1, green LED (G LED 3 W, Juxiang, China); 2, optical fibers (CORE200UM, Shouliang, China); 3, XYZ axial adjuster (XYZ25MM, Juxiang, China); 4, mechanical elements for supporting; 5, CMOS image sensor (DMM27UJ003-ML, The Imaging Source, Germany). The G LED’s claimed wavelength is 550 nm, and the spectral bandwidth is about 40 nm. In order to improve the spatial coherence, the LED light is coupled into an optical fiber whose core diameter is 200 μm. As shown in Fig. 1(b), the spatial-coherence-improved light departs from the end of the optical fiber at the top of the setup and then illuminates the bio-sample below. Then, the light diffracted by the bio-sample is harvested by the CMOS as a hologram. The distance between the end of the optical fiber and the bio-sample slide is about 100 mm. The distance between the CMOS and the bio-sample is less than 2 mm. The bio-sample is put in a mechanical holder, which is fixed on an XYZ axial adjuster with micrometer-level precision. In the process of the experiment, we should record three holograms. At the initial diffraction distance, we can get the first hologram. As shown in Fig. 1(a), the bio-sample is then moved about 50 μm along the Z axis, and the second hologram can be obtained. Finally, in order to harvest the third hologram, the bio-sample keeps on moving about 50 μm along the same Z-axis direction. The accurate diffraction distance can be computationally determined by the digital autofocusing method.
Autofocusing. Generally, if the defocusing range is small, the defocusing can be recognized as a Fresnel propagation because the LED illumination is partially coherent[11]. Distance autofocus algorithms can be used to calculate . The essential part of the computational autofocusing algorithm is iterative forward/back propagation based on a single digitally recorded hologram[12–
Phase retrieval algorithms. We adopt TIE and G-S phase retrieval algorithms to reconstruct in-focus image from the three holograms mentioned above[1–

Fig. 2. Computational algorithm flowchart to get a virtually colorized lens-free on-chip microscopy image.
Virtual colorization by deep learning. In this paper, the YCbCr color space is used for colorization. As shown in Fig. 3, there are two main parts to form a GAN, one is a generator network (GN), and the other is a discriminator network (DN). The GN’s architecture adopted by us is symmetric and added with skip connections, which is usually called U-Net.[5
Figure 4 is the experimental results of virtual colorful lens-free on-chip microscopy by our deep learning style transfer method. Three diffraction holograms at different defocusing distances are harvested by the CMOS. The complex wave-fronts are reconstructed by combining the TIE and G-S iterations phase retrieval method. Then, by training GAN with 200 pairs of the reconstructed amplitude images and conventional microscopy images (256 × 256 pixels), the deep learning style transfer kernel network parameters are obtained. When a new grayscale lens-free on-chip microscopy image is input into the trained GAN, a virtual colorful H&E stained microscopy image can be received. In hardware, the G LED (JXLED3WG, Juxiang, China) is with a spectrum bandwidth of at the dominant wavelength of 550 nm. The CMOS image sensor is MV-CB120-10UM-B/C/S, Hikvision, China, and the XYZ axial translation stage (XR25C/M, Zhishun, China) is used to align the sample and the CMOS image sensor. The GAN was implemented using TensorFlow framework version 1.4.0 and Python version 3.7. We implemented the software on a desktop computer with a Core i7-7700K CPU at 4.2 GHz (Intel) and 64 GB of RAM, running a Windows 10 operating system (Microsoft). The network training and testing were performed using dual GeForce GTX 1080Ti GPUs (NVIDA). The training time is , of which the virtual colorizing (image-style transferring) time of a lens-free image in practice or the test mode is . Three regions of interest (ROIs) are marked in Fig. 4, which are also zoomed in Fig. 5. Comparisons of lens-free on-chip microscopy images, bench-top commercial microscope images, and virtual colorization H&E stained images are presented in Fig. 5, which are image pairs of ROI #1, ROI #2, and ROI #3.

Fig. 4. Data processing to achieve virtual colorful lens-free on-chip microscopy. The yellow scale bar is 200 μm.

Fig. 5. Comparisons of lens-free on-chip microscopy image, bench-top commercial microscopy image, and virtual colorization image, which are image pairs of ROIs #1, #2, and #3 in Fig. 4 .
From the lens-free on-chip microscopy images of Fig. 5, under the G LED illumination, it can be observed that the cell nuclei are almost black, which should be stained by the hematoxylin dye with blue or purple, and other cellular matrix and cytoplasm parts are tintedgray, which should be stained by the eosin dye with pink color. These corresponding stained colors are also presented in the bench-top commercial microscope images in Fig. 5. In contrast, we also show the virtually colorized lens-free on-chip microscopy images by our proposed deep learning style transfer method. It can be seen clearly that the texture details of the tissue in our virtually colorized images are very well kept. Moreover, by our method, the black cell nuclei in the original grayscale lens-free on-chip images are virtually colorized as the bluish-purple color and the tinted gray parts are colorized as pink color simultaneously. The nuclei, cytoplasm, and boundaries of a tumor tissue slide could be recognized and differentiated easily and clearly in a colorful mode by our process. Figure 5 shows the largest advantage of our method is that, by our deep learning virtual colorization, only one quasi-chromatic illumination and a monochromatic CMOS image sensor would achieve visually colorful microscopy. The results are approximately the same with the true colorful images.
In this paper, we report a deep learning style transfer method to achieve virtual colorization images for H&E stained pathological diagnosis using the grayscale lens-free on-chip microscopy with only one chromatic illumination. The convincing experimental results demonstrate that our method works well and transfers the grayscale lens-free on-chip microscopy image to a colorful human vision image without any more data and hardware cost. It is believable that our method can be useful for improving the application of the lens-free on-chip microscope in telepathology and resource-limited situations.
[1]
[2]
[3]
[4]
[5]
[7]
[8]
[10]
[11]
[12]
[13]
[14]
[15]
[16]
[17]
[18]
[19]
[20]
[22]
Hua Shen, Jinming Gao. Deep learning virtual colorful lens-free on-chip microscopy[J]. Chinese Optics Letters, 2020, 18(12): 121705.